Physiological earthquakes: Predicting cardiac events combining seismic experience with AI in the hospital
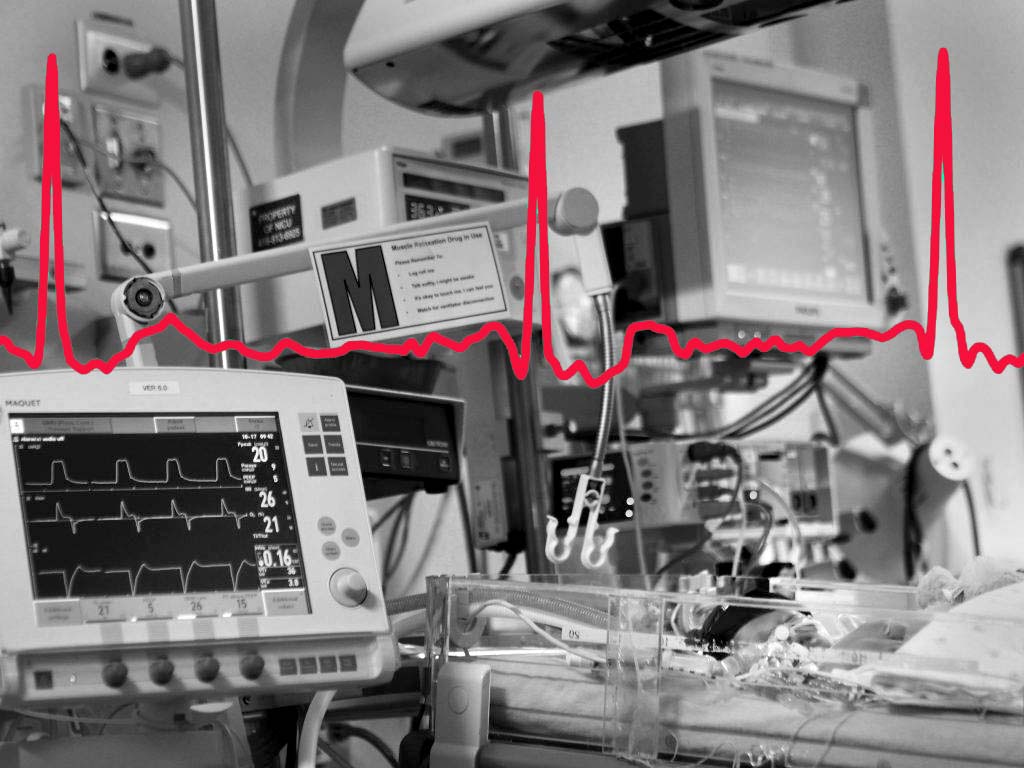
It is rare for a mineral engineering professor to receive funding from the Canadian Institutes of Health Research (CIHR), as it usually falls to biomedical engineering research and others directly involved with health care.
Professor Sebastian Goodfellow, from the Lassonde Mineral Engineering Program, with some CivMin graduate students and a team of predominantly medical doctors at The Hospital for Sick Children (SickKids), affiliated with the University of Toronto, have achieved exactly this. They’ve been awarded a $657,900 grant from CIHR to fund their research into deploying artificial intelligence (AI) in the intensive care unit (ICU) at SickKids to detect and diagnose heart arrhythmias.
Up to 29 per cent of critically ill children experience arrhythmias that cause deterioration and even death. These arrhythmic complications affect roughly 700 critically ill children at SickKids each year. Preventing these complications requires timely detection and accurate heart rhythm diagnosis, which is currently done by continuous clinician surveillance of single-lead electrocardiograms (ECG) displayed on patient monitors. Differing clinician expertise and experience in this task lead to errors and delays in detection and diagnosis associated with preventable patient harm.
Prof. Goodfellow, along with Dr. Mjaye Mazwi and the team at Laussen Labs, a multidisciplinary research group at SickKids, are working to develop and deploy a highly accurate AI capable of expert classification heart rhythm to prototype what they believe will be a generalizable solution to addressing the translation gap in AI for health care.
We met (virtually) with Prof. Goodfellow to talk about the plans for deploying this AI system and how it’s not just as simple as training a model.
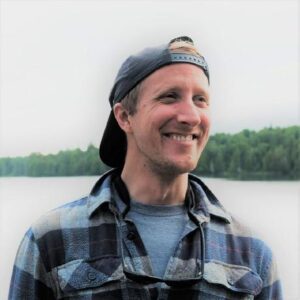
You were recently told you have been awarded funding through the Canadian Institutes of Health Research (CIHR).
Yes, along with co-PI [principal investigator] Mjaye Mazwi and the team at Laussen Labs, we have applied many times – perhaps this is the fourth or fifth time – and we’ve been close. In 2020 we were ranked 7th, but funding went to the top six. Again, in 2021 we were ranked 11th, when funding went to the top 10. This time we were successful and it’s very gratifying.
Usually someone in the medical field would receive this kind of funding. It’s unusual for the CIHR to award something engineering related, right?
Well, yes, it is strange. Always raises a few eyebrows. What does mineral engineering have to do with health care? On the surface, not much.
I joined Laussen Labs in 2017 to bring my signal-processing expertise to the group. My PhD research, which I conducted in our Department, focused on applied seismology, which is the study of seismic waves generated by engineering processes such as mining. At the time, Laussen Labs had just started acquiring physiologic waveform data, such as ECGs, which are the electrical signal of the heart. The analysis and modelling of high-frequency time series data require a skillset called digital signal processing. When analyzing earthquake seismograms during my PhD, and afterwards in the private sector, I acquired this skill set, which is how I first got into the health-care field.
However, these multidisciplinary teams are more common than you may think and the reason is the important problems of today and tomorrow spill across borders, cultural divides and fields of knowledge. For example, Laussen Labs developed a bespoke time-series database for the storage of physiologic waveform data at SickKids. The lead database architect was a hydrologist by training whose previous experience was developing a database for the storage of drone photography for a flood plane mapping application. There are, of course, many doctors in Laussen Labs but also computer scientists, a seismologist, a cognitive psychologist and yes, a hydrologist.
Over time, the gap between AI in mineral engineering and AI in health care has become smaller and smaller for me. Beyond publishing proof-of-concept studies in academic journals, deploying AI models in the real world is very hard and the challenges span mineral engineering, health care, and beyond.
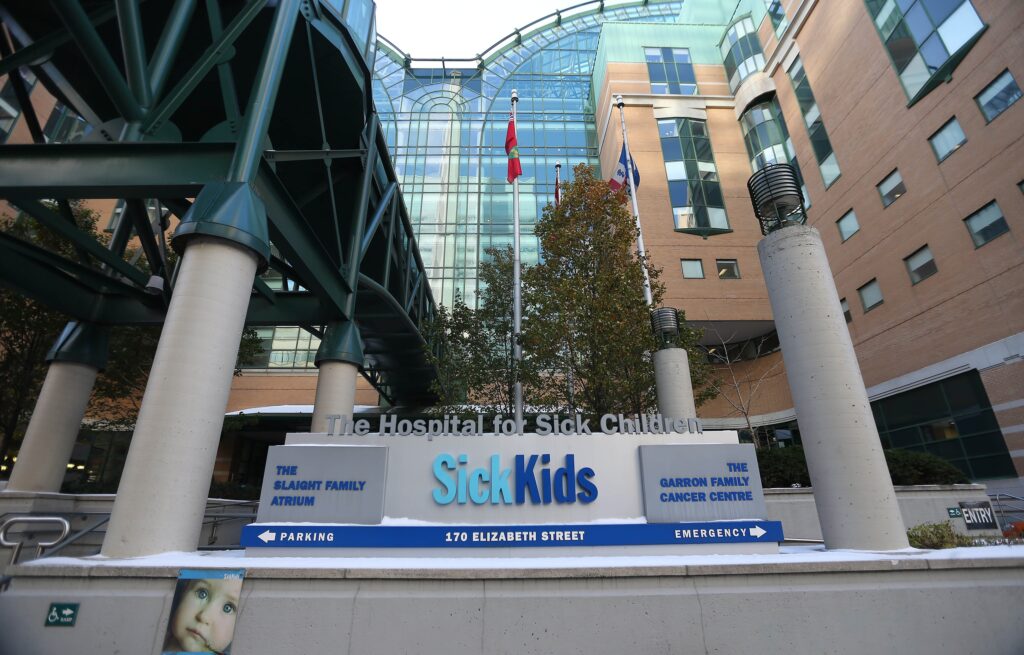
What is it you’ve proposed? And what will you do to fulfill this grant?
We are building and deploying a model that detects and diagnoses common pediatric heart arrhythmias using continuous ECG data, which is generally a task staff physicians in the ICU can do very well. The challenge is there are only two staff physicians on duty at any given time to service 42 ICU beds, and detecting and diagnosing heart arrhythmias is just a small part of their job. As a result, these arrhythmias often go undiagnosed for a period and the longer the delay, the worse the outcome for the patient. The idea is to use our expert clinicians to train an AI, which can match their performance and monitor all ICU beds 24 hours a days, seven days a week, looking for arrhythmias.
The model is actually quite vanilla. AI experts from the Vector Institute would be rather underwhelmed – it’s just a WaveNet model performing multiclass classification. Big deal, right? But, employing the golden rule of engineering, Keep It Simple Stupid (KISS), was deliberate. If you search the keywords AI/ Machine Learning/ Deep Learning + Health care in Google Scholar, you’ll find hundreds of thousands of academic papers and the growth is exponential. It’s a hot topic to say the least. However, if you dig a little deeper to see how many of those AI models actually made it to clinical deployment, it’s less than 0.1 per cent. We call this the “translation gap” and we made the decision to keep our model simple, so we could focus on translation.
The translation gap is a result of multiple factors. These include difficulties creating computational infrastructure that can reliably ingest data for real-time classification, the requirement of a production-grade Machine Learning Operations [MLOps] platform for serving, monitoring, and re-training AI models, regulatory challenges integrating AI models into clinical domains, and concerns about responsible validation and bias, sometimes described as “algorithmic fairness”. The team that can close this gap must include a wide range of expertise including bio-ethics, MLOps, law, cloud, software development, human factors, cognitive psychology, digital signal processing and machine learning.
VIDEO: This animation shows an ECG signal transitioning from a normal rhythm to an arrhythmia. In the top right corner is the model score for a particular pediatric arrhythmia called Junctional Ectopic Tachycardia (JET). When the signal transitions, you can see the model score increase.
You have a background in AI, correct?
Before joining U of T, I was the AI Lead at a startup in the mining industry called KORE Geosystems. We developed an AI product that automated various parts of geotechnical and geological core logging workflows. For example, rock type classification and fracture counting. In this role I had to deploy AI models that geoscientists relied on to do their work. This is where I learned just how hard deploying AI models in the real world is. I was able to bring this experience to Laussen Labs where they were running up against similar challenges.
When you’re building products, you’re forced to start from the business requirements and work backwards to the technical solution. Because products are built for users, it’s no surprise why this is the preferred approach. In research, it’s more common to start from a dataset, build a model, publish a paper, and then start thinking about the application, which is no wonder less than 0.1 per cent of AI studies make it to deployment. The product development mindset I developed in the private sector has been invaluable in successfully translating AI models into complex clinical environments.
I’ve sometimes heard people say they don’t trust AI. Is this going to be a challenge?
Trust is always a challenge when introducing any new technology into an established workflow, and AI is no exception. In health care and mining, AI adoption has been seriously impeded because of early and very public projects that started with building a model and only involved the end user, such as a doctor or a geologist, at the very end if at all. This model-centric approach gets people’s walls up quickly and we’re still trying to overcome it even to this day. Therefore, it is imperative to think about your AI model as a product from the very start, which will involve those end users in documenting requirements and ultimately build trust.
There is also a sci-fi perception of AI perhaps resembling Skynet from the Terminator movies. Whenever talking about AI with doctors or geologists, I always try to use the most boring descriptions I can think of. My favourite at the moment comes from the Head of Decision Intelligence at Google, Cassie Cozirkov, who describes Machine Learning, which is a subdomain of AI, as a “thing labeller”. The “thing” could be a photo of an intersection and the “label” could be the number of pedestrians in the intersection. For our arrhythmia model, the “thing” is a five-second segment of ECG data and the “label” is whether an arrhythmia is present. What’s all the hype about, right?
Lastly, how we present the performance of a model to the end user is important and, in my opinion, is the best way to promote trust. We need to use metrics that map to clinical key performance indicators, and we need to present those metrics in a transparent manner over long periods. Most people have no clue how a plane achieves flight or how a jet engine works but they feel safe flying. The reason is there is a one in 20 million chance of dying in a commercial airline plane crash. So, an arrhythmia model that is consistently performing at the level of a board-certified cardiologist will build trust.
By Phill Snel
Team members:
Mazwi, Mjaye, SickKids (co-PI)
Goodfellow, Sebastian (co-PI)
Assadi, Azadeh, SickKids
Bulic, Anica, SickKids
Ehrmann, Daniel, SickKids
Eytan, Danny, SickKids
Goldenberg, Anna, SickKids
Goodwin, Andrew, SickKids
Greer, Robert, SickKids
McCradden, Melissa, SickKids
Gallant, Sara, SickKids
Gnatenko, Vladislav (CivMin, MASc candidate)
Shubin, Dmitrii (CivE MASc 2T1)