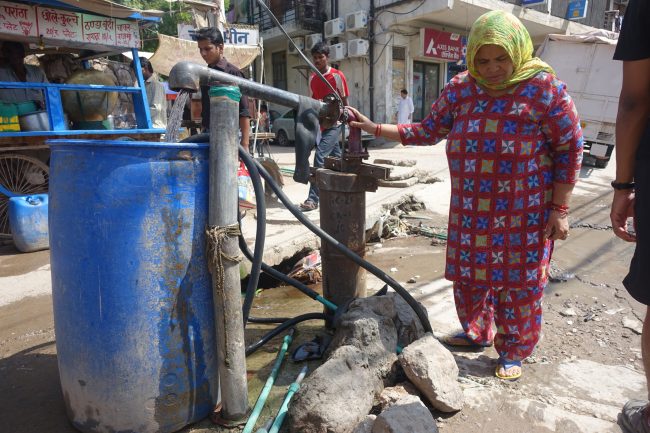
A multidisciplinary team from across the University of Toronto is developing tools and metrics to empower water planners, communities and activists, and improve water equity in India.
The project is led by Professors David Meyer (CivMin, CGEN), Nidhi Subramanyam (Geography & Planning) and Carmen Logie (Social Work). It is one of 17 to receive Research Catalyst Funding Grants through the Data Sciences Institute (DSI) at U of T.
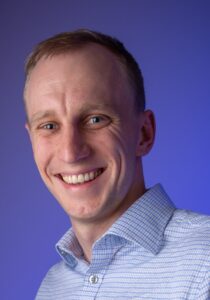
While India has made gains in expanding access to water distribution networks, rapid urbanization and inadequate infrastructure have resulted in systems that provide water for less than four hours per day in some regions, impacting 390 million people.
To cope, residents invest in water storage infrastructure and seek alternative water sources, imposing significant financial, environmental, educational, health and time costs, especially on women and girls.
“The level of complexity and inequality is staggering,” says Meyer. “Since 2018, I have been exploring options for visualizing and learning from water supply schedules.”
With their project, “Harnessing Data to Visualize and Mitigate Urban Water Inequities within the Cauvery River Basin, India,” Meyer, Subramanyam and Logie bring together diverse disciplinary perspectives on water and data — including a deep understanding of water engineering, water governance and equity.
“Water is a cross-cutting theme that flows through so many disciplines, geographies, technologies and aspects of our lives,” says Subramanyam. “Any study about water must engage different kinds of data, expertise and knowledge systems.”
“Professor Subramanyam has helped me realize that data cannot be interpreted properly without considering the data generation process and the data generators themselves,” adds Meyer. “Understanding the context and incentives for water utilities to report and curate data will prove key to leveraging it on an ongoing basis.”
All projects supported by the Catalyst Grants fund multidisciplinary research teams focused on using the development of new data science methodology or the innovative use of data science to address questions of major societal importance.
“The Data Sciences Institute is committed to fostering new opportunities to cultivate multi-disciplinary collaborations between data science methodologists and researchers in various application domains. This is just the beginning,” says Professor Timothy Chan (MIE), who is the DSI associate director of research and thematic programming.
“With this inaugural round, we received 70 highly competitive proposals, which were carefully assessed by a multidisciplinary review panel.”
The Data Sciences Institute Catalyst Grants are supported by the University of Toronto Institutional Strategic Initiatives and external funding partners, with two of the 2022 Catalyst Grants co-funded by Medicine by Design directed to finding solutions to challenges in regenerative medicine.
From U of T Engineering, the list of grant recipients also includes the following projects:
Preventing a Reproducibility Crisis in Quantum Computing: Benchmarking Quantum Computing Against Classical Algorithms for Molecular Property Predictions
- Hans-Arno Jacobsen (ECE) and Ulrich Fekl (Chemical and Physical Sciences, UTM)
Bioimage Informatics for Exploring Heterogeneous Cell Communities and Accelerating the Development of Effective Cancer Treatments (Project co-funded by Medicine by Design)
- Joshua Milstein (Chemical and Physical Sciences, UTM), Alison McGuigan (ChemE) and Rodrigo Fernandez-Gonzalez (ChemE, BME)
Predicting And Preventing Chronic Disease burden in populations (PREPARED): Deploying decision-support tools for the prevention of chronic diseases
- Laura Rosella (Dalla Lana School of Public Health), Birsen Donmez (MIE), Alfred Myrtede (MIE), Hailey Banack (Dalla Lana School of Public Health) and Greg A. Jamieson (MIE)
Reduce Early Revisions of Joint Replacements through Data Science Strategies
- Yu Zou (MSE), Qian Sun (Computer & Mathematical Sciences, UTSC) and Adele Changoor (Lunenfeld-Tanenbaum Research Institute)
By Data Sciences Group
This Story originally published by Engineering News