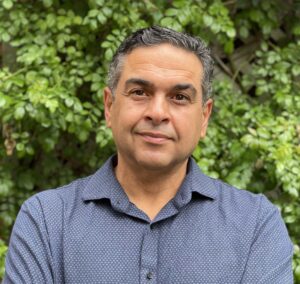
Tamer El-Diraby
Professor
Department of Civil & Mineral Engineering
Email: tamer.diraby@utoronto.ca
Office: GB329
Tel: 416-978-8653
Expertise:
Areas of Specialization:
Construction Project Management
Background
The goal of my research is to use informatics systems to bring about fundamental shifts in the manner used to design and manage construction projects. I use machine learning and data management tools to explore new horizons in project management, particularly to empower communities to innovate new sustainable environments. The aim is to shape the smart city as sustainable and adaptively responsive.
Knowledge capture and re-use (socio-semantic analytics): how can we use advances in informatics to facilitate collaborative generation and use of knowledge?
Asset management: how can operational policies and rehabilitation decisions make facilities smarter, more sustainable and achieve higher levels of services?
Smart city and regenerative sustainability: Can we make urban projects a conduit for innovation democratization and empowerment: citizens co-creating new solutions for climate action not only through configuring the physical aspects of the project but also through collaborative behavior change and supportive, community-driven operational policies.
- Construction project management
- Informatics & knowledge management
- Infrastructure asset management
- Building information modeling (BIM)
Education and Designations
BSc., MSc. (Zagazig, Egypt)
Ph.D. (Texas)
- Affiliate + Teaching and Learning Sub-Committee Member - President's Advisory Committee on Environment, Climate Change and Sustainability (CECCS)
- Affiliate - U of T Data Sciences Institute Network (DSI)
- Affiliate - U of T Mobility Network
Affiiate - University of Toronto Transportation Research Institute (UTTRI) - Affiliate - FASE Centre for Analytics and AI Engineering
- Affiliate - FASE Institute for Sustainable Energy
Research Agenda
The core of my research work focuses on knowledge and informatics systems in construction project management. To me, the time horizon of a project covers its whole life cycle. I try to expand the analysis beyond the planning and construction to include operations and asset management phase. Furthermore, projects should blend with its wider urban context. The planning and operations of urban projects must address community needs, promote sustainability, and enhance overall resiliency. A core element of my work focuses on stakeholders’ management. This is not limited to engaging and serving project teams (public officials or professionals). Citizens are equal partners, not only in decision making, but also in co-creating solutions. This is because I view knowledge as an evolutionary social phenomenon: it emerges through interactions between networks of people, with citizens as a valid, in fact, the original, source of innovation. To this end, I embrace a pragmatic epistemology that aims to balance the dynamics of bottom-up (constructivism) with the formality of top-down for discovery and modeling of knowledge.
In modeling knowledge, I conduct case studies to capture lessons learned into re-usable decision support systems. These, along with input from experts, are formalized into ontologies. In parallel, I utilize social media and other means to reach out to and profile citizens groups, ideas, and opinion dynamics. I then use semantic and social network analysis tools to both extract and share knowledge from/with all stakeholders. To this end, I try to link these tools to building information models (BIM) to facilitate interaction and the co-creation of green designs and operational policies. I use machine learning to help (citizens and) decision makers discover patterns in the unstructured data of projects (documents and social interactions).
I am expanding the scope of my research to consider the future of smart cities. Hardware is not the fundamental challenge for smart cities. Data and behavior change are—especially if we want to make our city sustainable and more resilient. I am starting to explore integrating virtual reality gaming in project scoping and citizen engagement. The focus is to make these games adaptively interactive to foster co-creation of knowledge and support the development of shared vision and objectives of projects and operational policies. To help enrich the dialogue, I use social and semantic network analysis to capture and feedback generated ideas or values into the gamming (innovation) exercise. I plan to work on complementing this with the use of cognitive and anticipatory computing tools to help find, distill and deliver relevant big data to stakeholders based on their profiles and the nature of ideas and values they are interested in.
The ultimate aim is to transfer the use of construction knowledge and artificial intelligence in the smart city from a decision making tool into an environment for promoting collaboration and making urban project means for propelling sustainability, community empowerment, and innovation democratizations.
My work can be seen as spanning two main domains of knowledge analysis and management systems: semantic modeling and data analytics. The work is applied to three main domains: Social BIM and intelligent buildings, Asset management, and Smart city systems
This thrust of research work represents the core of my research contribution to construction informatics. I started working on developing ontological models for construction and infrastructure systems in 2002. My work in this area was amongst the earliest application of this approach in construction and, now, one of the most comprehensive. Traditionally, modeling work in construction focused on the classification of products and, later, product data models (such as IFC) as well as standardized models of process data. My work advocated two major shifts. First, focusing on semantics rather than data modeling. By virtue, this is the core objective of ontology. Second, and more uniquely, emphasizing the role and need to model actors in addition to products and processes. This is not just limited to project players [P12, P23], but also non-professional stakeholders such as members of the community [P19]. This stems from my belief that knowledge is a social phenomenon that emerges within networks of human beings. Later, this approach enabled me to be one of the early contributors in the formalized use of social network analysis in capturing and re-using knowledge embedded in social dialogue in project management practices [P38, P40, P44].
An informatics ontology is a formal description of what we know about a domain of discourse. It includes hierarchies (taxonomies) of concepts, their relationships and the axioms (limitations) that describe their behavior. Informatics ontologies interact very well with data standards to support interoperable computer programs as well as human interpretation (linguistic-savvy). While ontologies are related more to knowledge representation (rather than reasoning), they are normally used to complement AI tools to enhance their decision support capabilities.
With my students, I developed a set of ontologies to represent knowledge with the underlying belief that knowledge representation should balance the need for reasoning and the needs for communication to make decisions. This included developing ontologies for urban infrastructure products [P30], processes in infrastructure and construction domain [P27], actors and roles [P19, P23], green construction [P24], and finance of privatized infrastructure systems [P15]. These ontologies were used to develop AI-enabled web-based systems for corporate memory [P12]; process integration in virtual organizations [P26]; integrating sustainability in the routing of urban utilities [P29]; and social semantic communication in construction [P10].
Currently, the ontology network encompasses 4,000 concepts. It was the core semantic model in two major Canadian research projects. The first, funded by JIIRP (Joint Infrastructure Interdependency Program), which aimed at creating an interoperable understanding of the concepts of risk and interdependency across urban infrastructure systems [C52]. The second, funded by CANARIE, established an online, real-time platform for integrated management of intelligent transportation systems (ONE-ITS). The expertise of these project enabled me to develop the first domain ontology for construction (DOCK) [P36].
My current work in this research thrust focuses on the use of linked data systems to create interoperability between ontologies and other data standards. This is because no one ontology will be able to present all the concepts and needs of the AEC domain. Equally important, we need to link AEC ontologies with those of other related domain to meet the expanding (business integration) needs of knowledge organization. At the ontological level, I developed an approach for margining ontologies using lattice algebra [P28]. At the data integration level, the Green 2.0 project updated IFC to enable the use of social network analysis. Our work went beyond the scope of BFC to consider the semantics of comments and their networks [P42]. More importantly, actors and their networks are an essential part of the linkage process. In other words, we combine data of the physical products with the semantics of their context and the actors who are generating the knowledge.
Epistemological analysis of construction informatics
While ontology represents what we know about a domain, epistemology debates how (by what means) do we know what we know. Fundamentally, analysis of informatics epistemology is an attempt to explore, discover and possibly develop (new) theories for knowledge in the domain. This is needed given the drastic impacts of the proliferation of communication technology in the society and within the AEC domain. Of particular interest are the advancement in semantic and social web technologies.
My work on (questioning) the epistemology of construction informatics developed a proposal for complementing the dominant positivism with constructivist, bottom-up, discovery of knowledge [P32]. Such approach puts people at the center of knowledge creation and capture. It also emphasizes the dynamic nature of knowledge: local context of the project and community has a major impact on configuring the structure of knowledge. This proposed approach was extended into three major dimensions. First, customizing ontology development and management to the needs of the construction domain. I work on benchmarking lessons learned in designing ontologies—how can we balance the mechanics and standardized nature of ontology modeling (through OWL—Web Ontology Language) with the needs for representativeness, flexibility (customization), and usability. At the theoretical level, I proposed using the Extended Mind Theory for configuring ontology design to be more efficient, domain-specific and futuristic [B4]. At the pragmatic level, I developed a guide for validating ontologies based on three dimensions: epistemology (assumptions made), consistency (through using first-order logic), and communication adequacy (linguistic relevance) [P34].
Second, in addition to the top-down and formal development of knowledge models, the increasing role of big (unstructured) data presented an opportunity (and requires us) to explore more bottom-up and dynamic capture of knowledge. We are exploring the use of Blockmodeling to analyze semantic networks of project documents to extract knowledge constructs [P50, P51]. While each project is unique, they all share a common mosaic of concept. What makes a project unique is the set of (mosaic) concepts contained in it and, more importantly, their relationships. This approach is constructivists (discovers knowledge as expressed by the participants); dynamic (accumulate knowledge every time a document is added), context-sensitive (not a standard model, but concept blocks from within the project); and, more importantly, networked (we compare blocks of concepts in the form of a network, not just keywords or even a simple taxonomy).
Third in my interest is contemplating research methodology. As the needs and format of knowledge in construction informatics change, how can we upgrade and benchmarking research methods and validation [P5]? How can we handle the needs and challenges (sometimes, hype) of data analytics [P54]?
My work has focused on exploring the socio-technical nature of projects and, in particular, molding and integrating knowledge(s) of professionals and citizens. With the increased technical agency of stakeholders and the increasing power of knowledge analytics tools, we now have the ability to extract formalized knowledge constructs from stakeholders’ interactions [P23]. Unstructured data analysis can be a source for extracting knowledge from documents and stakeholder interactions. Lately, my interest and contribution in this field evolved into the use of social media in reaching out to communities [P38, P44, P47]. Beyond the engagement, my work over the last five years has focused on conducting an in-depth socio-semantic analysis of the contents and social networks of project discussion networks (PDN). (social) Network analysis is used for capturing knowledge in contrast to its traditional usage in construction research: study communication and organizational structures of projects. My student, Mazdak Nik Bakht, and I reviewed the PDNs for 80 of the top 100 infrastructure projects in North America; closely tracked 25 of them; and analyzed in-depth 4 of them. We developed the first extensive analysis of PDN. Instead of using off-the-shelf tools, we have developed and validated new algorithms specific to PDN [P40]. The socio-semantic platform includes several themes:
Contextualizing the definition of sustainability: Using a Game-With-A-Purpose (GWAP), this service exposes citizens to a set of already existing indices for sustainability [P49]. They are asked to classify input (such as tweets) created by other citizens over the PDN according to the categories in the indices. Analyzing their input helps to modify the structure of existing indices to reflect the needs/attitudes of the local community. Instead of using static indices or directly asking users for formal inputs, such a semi-structured approach will balance the need for professional rigor and, at the same time, will reflect context-sensitivity [P41].
Understanding PDNs within project context: the objectives of this service are twofold: semantic analysis of terms and concepts discussed by users over the PDN for clustering the ideas and topics discussed [P52]; and to study patterns of influence among end-users (nodes) [P44].
Analysis of opinion dynamics: nodes of a PDN express their opinion in the form of subject-sentiment dyads. Each discussion is composed of statements, and each statement discusses an aspect of the project (a subject) by supporting or opposing it (the sentiment) [P46, P52]. We study these dyads and their evolution as the participants’ social network changes.
Customized user communications: use recommender systems to customize communication to stakeholders based on their social and semantic profile. This service uses a publish/subscribe system to link each user to a set of project documents that matches their profiles (needs). In cases where no relevant project documents are available about the desired topic, the user will be directed to the relevant knowledge sources on the web (especially Wikipedia) [P47].
Capturing knowledge constructs: This service will explore the usage of the material provided to users and will analyze the links/similarities between them to detect concept patterns [P51]. This is to be used to extract knowledge constructs from the unstructured data of users and their input/documents [P50]. Collating these constructs will help discover a common concept mosaic (a bottom-up ontology).
Data reliability and collection features high in this thrust. We studied subsurface utility engineering (SUE) role (and return on investments) in enhancing data reliability [P22]. Similarly, we analyzed the life cycle costs of smart infrastructure systems to justify and optimize investments in real-time data collection [P4].
My group also works on structured data analytics. Currently, we are using machine learning to help discover patterns of asset deterioration and efficiency of maintenance programs. We developed an interactive system and map to help municipal decision makers predict road conditions in the near future [P55]. The emphasis is to help municipalities (especially smaller ones) assess the gaps and investments needs and coordinate decision making [B5]. We are also using data analytics for studying means to analyze the impacts of climate change on asset deterioration [56].
As a culmination of our R&D into social and semantic systems, I led a major project (Green 2.0) supported by CANARIE (an R&D arm for the Ministry of Industry in Canada) to develop a middleware platform for integrated, multi-stakeholder, socio-technical analysis of green buildings (see Figure 3). Green 2.0 combined BIM with social network analysis and online, automated analysis of energy usage [P42]. The Green 2.0 platform was the first to embed unstructured and semantic user interactions into BIM. Unlike using BCF, Green 2.0 approach provides users with a seamless ability to exchange views and ideas in an open manner. Furthermore, BIM was linked to energy analysis software to evaluate energy usage in buildings. Project teams can study different project designs and, for each, they can estimate the expected energy usage. Providing access for such features to end-users is a major component in securing their support for greener options.
Green 2.0 takes BIM from the realm of a digital platform into the realm of a socially-based collaborative platform for decision making. We give people the controls of BIM software to suggest, choose, study and innovate new means to design and operate their facilities. Through publishing BIM to all stakeholders (professionals, public and business) we can harness the intelligence of the crowd.
Intelligent buildings:
A smart building has sensors. An intelligent building can learn and adaptively interact with its systems and users. Our work aims to engage users in analyzing energy consumption; understand their needs; collect their ideas, profile their usage of the building; and model their comfort levels. Using BIM, data analytics and anticipatory and cognitive computing, the SBEPA initiative is transferring six buildings at UofT to predict the energy and activity scenarios and negotiate with its users an optimal configuration for use and energy management schemes. In addition to the multidisciplinary research and the socio-technical of this project, it represents a chance for me to explore the future of BIM: how to transfer it from the realm of a collaboration and data interoperability environment, into an analytics platform. How can this (parametric) data-rich environment become an environment for knowledge management and “business intelligence”?
I am leading an initiative in buildingSmart International to formalize the business case for automated rule checking (ARC). The aim is to shift the debate about ARC in two directions. First, realizing the immense opportunity and value of ARC beyond its traditional limited role: speeding e-permitting. ARC that is based on machine learning is base for realizing and managing intelligent buildings, which is a more impactful application of ARC. Second, moving the analysis and decision making about ARC form quantifying its technical advantages (dollars saved due to error reduction) into its crucial rule as a linchpin for data-enabled AI in the industry. A small project with Sidewalk Labs is considering the use of ARC in real-time operations of energy and environmental systems in high-rise mass timber buildings.
My research in asset management focuses on the areas of decision support systems, data management and analytics, and organizational and policy making issues.
Organizational change
Aware of the essential role and need for a cultural change, we conducted a critical review of research work on decision making models over the last 50 years. We analyzed the modeling approach, AI tools used, nature of analysis parameters, as well as epistemological assumptions. We developed a framework for reconsidering the whole process of decision making in construction and asset management practices [P39]. The proposed approach promotes evolutionary, bottom-up discovery of knowledge and consensus building. Similarly, we are studying means to revamp pre-project planning (and environmental impact assessment) to expedite approvals while promoting engagement and sustainability [P24]. We present an implementation of the proposed decision making framework that balances the power between citizens and project proponents and promote the co-creation of project features and plans [P48].
I developed a competency assessment framework to help “change management” in municipalities. The aim is to enable efficient implementation of asset management beyond the limited scope of maintenance scheduling or even life cycle costing assessment [P45]. On a bi-annually basis, we run a survey with OGRA for assessing asset management practices and competencies in Ontario’s smaller municipalities. I am also engaged in the buildingSmart international work on expanding IFC to infrastructure systems.
Integrated decision making
With my student, Hesham Osman, I developed an ontology-based GIS system to coordinate the planning of urban infrastructure systems [P29]. The system analyzes the routing of urban water-mains through a three-dimensional semantic analysis. The first dimension represents the physical and the socio-economic attributes of the area under study. The second dimension represents the physical and sustainability features of the pipe system suggested. The third dimension represents the decision criteria, which can encompass features such as life cycle costing, impact on traffic, the impact of maintenance on nearby businesses, and community and environmental impacts. This included developing a new ontology [P30], and a platform to enrich GIS with semantic features [B2]. Other work by my students developed models and decision support systems to help integrate project planning and optimize construction work [P6, P14, P21].
ONE ITS project (funded also by CANARIE in 2007), represented a way to implement my vision of integrated semantic-based operations of infrastructure assets. The project developed an ontology for infrastructure interdependency, including modeling of threats, vulnerabilities, and risks [C52]. This was used to power a middleware engine to study roadway incidents and coordinate actions accordingly, including the dispatch of emergency personnel and reconfiguring traffic flow as needed.
To address the limitations and diversity of data in the asset management domain, I use machine learning to help process unstructured data. For example, working with colleagues in the transportation domain, we are using socio-semantic analytics of user satisfaction and travel patterns to guide the decision making in transit (mobility) projects [P46]. Linking usage patterns and project features is at the core of optimizing asset management decisions: which operational policies have the greatest impacts on levels of services? Which rehabilitation work will have the greatest impact on energy consumption? Which new projects will have the greatest impact on overall system efficiency? In essence, we expand the options pool: do we need a behavior change or a re-design of operational policies, or a rehabilitation of facilities, or to build a new one? Such considerations are at the core of any investment for (future) assets in the smart city, especially in relation to driverless cars [P53].
The next frontier in my research relates to the role of informatics and asset management in the city of the future. With the challenges of climate change and the opportunities of smart city, public agencies have to re-engineer their role to be more proactive and to lead a consorted effort to enhance the efficiency of urban systems, and levels of services [P13, P48]. At the core of this change is a paradigm shift in the role of citizens in the smart city. Lately, community objections have stopped or delayed many large projects—resulting in budget escalation. Using ontologies, analytics, and BIM, my students and I developed tools for customizing the delivery of information to project stakeholders [P10, P33, P47]. We developed means for profiling communities, their views, and the dynamics of their opinion as project scope and features change.
Socio-technical analysis of urban systems:
One key area of research in this area focuses on managing transit assets—as a precursor to investigating the management of AV systems and assets. In collaboration with colleagues in transportation, we used the same approach for profiling the ideas and satisfaction levels of transit users: what are the topics of interest, how do they change with group dynamics and how do these impact information diffusion and opinion making [P46, P53]. In addition to providing a more effective means for understanding and communicating with communities, this line of research is a step in creating models for the role and use of data in planning and operational decisions for smart systems (such as driverless cars). In this work, we highlight the future of project decision making: behavior change is integral in any project; prototyping as an interim step in realizing project values and impact; and the centrality of community approval to any new project [41, 43, 47, 49]. This example and others highlight the value of piloting in decision making in today’s socio-technical culture. In many cases, such physical prototyping will not be feasible. The next best thing is a data-rich and interactive virtual reality portal such as the one suggested here.
Regenerative sustainability:
FutureTalks is a research initiative at UofT based in the Munk School of Global Affairs, with collaboration from 30+ professors, NGOs, and public agencies, which aims to engage citizens in generating sustainable futures for Toronto. The objective is not just to develop a scenario or a plan. The aim is to study and develop an environment for empowering citizens to lead imagining futures for their city, co-developing policies and collaborating in decision making [P25, P37]. The result is a city with empowered citizens and more resiliency, especially in regards to climate change and the deployment of smart systems. The project will engage 100,000 citizens in Toronto through high-touch (face-to-face events), and high-tech tools. In the latter, which I lead, we plan to use interactive virtual gaming to help visualize scenarios; social network analysis to profile citizen groups; semantic analysis to capture ideas and opinion dynamics; and cognitive and anticipatory computing to find, customize and deliver relevant big data to citizens as they deliberate and innovate [P41, P43, P47].
Business intelligence and process automation.
This line of work is used in another project, with the Ministry of Transportation Ontario (MTO). The project aims to enhance the accuracy of preliminary estimates. We are using data analytics to support enhancing value engineering and constructability analysis and implementation of best practices. Equally important, the research is considering the use of such analysis as a linchpin for advancing data collection and management as well as the use of business intelligence and process automation in managing MTO projects.
Value engineering and constructability analysis are two of the most important competencies in AEC process. They have a direct and early impact on design optimization and construction efficiency. To help formalize the two processes, we developed a comprehensive value-based model for design optimization [P20]. In this work, we provided a new and unique model for capturing stakeholder values, analyzing different design options and their (miss)matches, and using an optimization technique (data envelopment analysis) to link the two concepts: what is valuable to users and what is technically feasible. The same approach was later used in the Green 2.0 model. In this case, however, we captured both user and professional preferences through analysis of the social networks and the semantics of their comments. The integration of these two systems can help in automating parts of the value and constructability analysis. With the help of machine learning, we can also capture patterns and provide business intelligence services to project stakeholders [50, 51].
AEC business models in the smart city
I have a firm belief in a two-way symbiotic relationship between informatics research and business trends. So, parallel to envisioning new definitions and futures of the city, I am working on studying the changes in AEC business models: where can the industry contribute; what are the opportunities for enhancing overall performance. For example, due to the increased complexity of projects and the growing role of the private sector in infrastructure development and operations, IPD is gaining momentum in the industry. Unlike the traditional needs (for schedule and budget optimization), practitioners need tools to manage information flows, broker diversified knowledge(s), and foster trust. An Equally important force for considering new directions in AEC business models is (the “pull” of) advances in data systems and artificial intelligence. BIM (Building Information Modeling) is creating interoperability and a data-rich environment for projects. Big data analytics and machine learning tools, if integrated into BIM and industry practices, afford us opportunities to expand our understanding and explore innovative futures to our practices.
To this end, I have developed a set of case studies on assessing traditional marketing decisions such as market attractiveness and competition levels [P16]. I am also very interested in the impacts of globalization on construction [P35]. Green 2.0 implements a BPM platform for integrating information and product supply chains. For this, we are re-using a semantic process synchronization mechanism, previously developed by one of my students [P26]. The aim is to embed business process management within BIM to coordinate the flow of tasks—based on the semantics of each process.
One of our projects aims to promote ARC R&D as the key for advanced knowledge management, process automation, and (indeed) organizational transformation.
The Centre is a node for industry-academia collaboration to enable the adoption of information technology in the construction industry. The Center’s main objective is to optimize project development through coordinating planning and operations across independent jurisdictions, nourishing a thriving information supply chain in the construction marketplace including the advancement of smart workplaces and intelligent infrastructure systems. For more details about the mission of the centre please visit the Centre's website
Funding for the Centre was secured through grants from CFI, OIT, UofT, and industry. Five overlapping tools have been identified to realize these objectives:
- Research: integrating deigns of collocated projects; coordinating construction plans; establishing collaborative virtual organizations; defining, measuring and integrating sustainability into the development process; modeling the needs and techniques for ecosystem restoration; establishing measures and tools to optimize public investments; benchmarking successful implementations in other industries and other countries; developing legal frameworks for e-infrastructure;
- Real-time data collection: a network of digital cameras, sensors, intelligent devices (such as smart gas valves, traffic signals, pollution monitoring stations, etc.) will be installed throughout Toronto. The Centre will integration data collected from all sorts (manually, sensor-based or network-based) into a harmonious repository of “infrastructure data” to support investment decisions.
- Software systems and web services: support a collaborative portal for integrating infrastructure design, coordinating construction, analysis of infrastructure inter-dependency, and optimizing public investments.
- Industry Forum: engaging industry to define research agendas, and validate/implement research results. Establish collaborative work environments, investigate impacts of public policy on the industry, and analyze strategies to benefit from globalization.
- e-society portal: engaging the community in infrastructure development through a web portal designed to solicit community input in the design of future infrastructure (including simplifying decision options for the public and building consensus).
Publications
B6. Piryonesi, M. and El-Diraby, T. E. (2018). Using data analytics for cost-effective prediction of road conditions: the case of pavement condition index, FHWA Report HRT-18- 065 (peer-reviewed); winner, ASCE/FHWA data analytics award
B5. Nik Bakht, M., and El-Diraby, T. E. (2015). Towards Bottom-up Decision Making and Collaborative Knowledge Generation in Urban Infrastructure Projects through Online Social Media. In Matei, S. A., Russell, M. G., Bertino, E. (Eds.) Transparency in Social Media--Tools, Methods and Algorithms for Mediating Online Interactions (pp. 145-164). Springer Publ., New York, NY.
B4. El-Diraby, T. E. (2015). From Deep Blue to Watson: the nature and role of semantic systems in civil informatics. In Issa, R., Mutis, I. (Eds.) Ontology in the AEC industry: a decade of research and development in architecture, engineering, and construction (pp. 97-120). ASCE Publ., Reston, VA.
B3. Nik Bakht, M., Kinawy, S., and El-Diraby, T. E. (2014). Construction Management and Public Participation. In Garrett, M. (Ed.). Encyclopedia of transportation: social science and policy, (Vols. 1-4). SAGE Publ., Thousand Oaks, CA.
B2. El-Diraby, T. E. and, Osman, H. M. (2009). Ontologies for linking CAD/GIS. In Karimi, H., and Akinci, B. (ed.) CAD and GIS Integration (pp. 171-181). CRC Press-Taylor & Francis Publishers, ISBN: 9781420068054.
B1. El-Diraby, T. E. (2006). Infrastructure development in the knowledge city. In Smith, I. (ed). Intelligent Computing in Engineering and Architecture (pp. 175-185). Springer, Berlin, Heidelberg.
P51 Aragao, R. R., and El-Diraby, T. E. (2019). “Using Blockmodeling for capturing project knowledge constructs: the case of energy analysis in the construction phase of oil and gas facilities”. Advanced Engineering Informatics, 39, 214-226.
P50 Aragao, R. R., and El-Diraby, T. E. (2019). “Using network analytics to capture knowledge: three cases in collaborative energy-oriented planning for oil and gas facilities”. Cleaner Production, 209, 1429-1444
P49 Nik Bkht, M., Hosseini, M., and El-Diraby, T. E. (2018). “Game-based crowdsourcing to support collaborative definition of sustainability in green projects”. Advanced Engineering Informatics, 38, 501-513.
P48. Bucci, M., and El-Diraby, T. E. (2018). “The functions of knowledge management processes in urban impact assessment: the case of Ontario”. Impact Assessment and Project Appraisal, 36(3), 265-280.
P47. Kinawy, S., El-Diraby, T. E., and Konomi, H. (2018). “Customizing information delivery to project stakeholders”. Sustainable Cities and Society, 38(4), 286-300.
P46. Hosseini, M., El-Diraby, T. E., and Shalaby, A. S. (2018). “Supporting sustainable system adoption: socio-semantic analysis of transit rider debates on social media”. Sustainable Cities and Society, 38(4), 123-136.
P45. Ismaili, D., and El-Diraby, T. E. (2017). “Organizational competency in urban water infrastructure asset management”. Canadian J. of Civil Engineering, 44(12), 1056-1070.
P44. Nik Bakht, M., and El-Diraby, T. E. (2017). “Project collective mind: unlocking project social discussion networks”. Automation in Construction, 84(12), 50-69.
P43. Kinawy, S., Nik Bakht, M., and El-Diraby, T. E. (2017). “Knowledge mismatches in stakeholder communication: the case of the Leslie and Ferrand transit stations, Toronto, Canada”. Sustainable Cities and Society, 34(10), 239-249.
P42. El-Diraby, T. E., Krijnen, T., and Papagelis, M (2017). “Green2.0: integrated BIM-based analysis of green buildings”. Automation in Construction, 82(10), 59-74.
P41. Nik Bakht, M. and El-Diraby, T. E. (2016). “SUSTWEETABILITY: a social annotation game for exposing public community's perspective on sustainability of urban infrastructure”. International J. of Human-Computer Studies, 89. 54-72.
P40. Nik Bakht, M., and El-Diraby, T. E. (2016). “Communities of interest–interest of communities: social and semantic analysis of communities in infrastructure discussion networks”. Computer-Aided Civil and Infrastructure Engineering, 31(1), 34-49.
P39. Nik Bakht, M., and El-Diraby, T. E. (2015). “Synthesis of decision-making research in construction”. Construction Engineering and Management, 141(9), 04015027.
P38. Nik Bakht, M., and El-Diraby, T. E. (2014). “Hidden social networks that drive online public involvement in infrastructure construction: case study of light rail transit projects in North America”. Transportation Research Record, TRB, #2453/Planning.
P37 El-Diraby, T. E. (2013). “Civil infrastructure decision making as a chaotic sociotechnical system: role of information systems in engaging stakeholders and democratizing innovation”. Infrastructure Systems, 19(4), 355-362.
P36. El-Diraby, T. E. (2013). “A domain ontology for construction knowledge”. Construction Engineering and Management, 139(7), 23-34.
P35. Cariaga, I., and El-Diraby, T. E. (2013). “Marketing Canadian housing construction products in Mexico”. Construction Engineering and Management, 139(6), 1-9.
P34. El-Diraby, T. E. (2013). “Validating ontologies in informatics systems: approaches and lessons learned for AEC”. Information Technology in Construction (ITcon), 19(28), 474-493.
P33 Zhang, J. and El-Diraby, T. E. (2012). “Social semantic portal for coordinating construction communication”. Computing in Civil Engineering, 26(1), 90-104.
P32. El-Diraby, T. E. (2012). “Epistemology of construction informatics”. Construction Engineering and Management, 138(1), 53-65.
P31. Ballan, S., and El-Diraby, T. E. (2011). “A Value map for communication systems in construction”. Information Technology in Construction (ITcon), 16(44), 745-760.
P30. Osman, H., and El-Diraby, T. E. (2011). “An ontology for construction terms in utility products”. Automation in Construction, 20(8), 1120-1132.
P29. Osman, H., and El-Diraby, T. E. (2011). “A knowledge-enabled system for routing utility infrastructure in urban areas”. Construction Engineering and Management, 137(3), 198-213.
P28. El-Gohary, N., and El-Diraby, T. E. (2011). “Merging architectural, engineering and construction (AEC) ontologies”. Computing in Civil Engineering, 25(2), 109-128.
P27. El-Gohary, N., and El-Diraby, T. E. (2010). “A domain ontology for processes in infrastructure and construction”. Construction Engineering and Management, 136(7), 730-744.
P26. El-Gohary, N., and El-Diraby, T. E. (2010). “A dynamic knowledge-based process integration portal for collaborative construction”. Construction Engineering and Management, 136(3), 316-326.
P25 El-Diraby, T. E. (2009). “E-city Knowware: knowledge middleware for coordinated management of sustainable cities”. Design Management and Technology (Gestão & Tecnologia de Projetos), Brazil 4(2), 3-25.
P24. Surahyo, M., and El-Diraby, T. E. (2009). “A knowledge management system for the environmental costs of highway construction”. Construction Engineering and Management, 135(4), 254-266.
P23. Zhang, J. and El-Diraby T. E. (2009). “SSWP: a social semantic Web portal for effective communication in construction”. J. of Computers, 4(4).
P22. Osman, H., and El-Diraby, T. E. (2007). “Implementation of subsurface utility engineering in Ontario: cases and a cost model”. Canadian J. of Civil Engineering, 34(12), 1529-1541.
P21. Osman, H., El-Gohary, N., and El-Diraby, T. E. (2007). “Integrating value engineering and context sensitive solutions: the case of St. Clair Ave. West transit improvements project". Transportation Research Record, 2025, 81-89.
P20. Cariaga, I., El-Diraby, T. E., and Osman, H. (2007). “Optimizing project scope based on life cycle costs and value achievement: the case of smart educational facilities”. Construction Engineering and Management, 133(10), 761-770.
P19. El-Gohary, N., Osman, H. and El-Diraby, T. E. (2006). "Stakeholder management for public private partnership". Int’l J. of Project Management, 24(7), 595-604.
P18. Osman, H., and El-Diraby, T. E. (2006). “Ontological modeling of infrastructure products and related concepts,” Transportation Research Record No. 1984, Highway Facility Design.
P17. El-Diraby, T. E., Lima, C., and Fies, B. (2006). “Strategies for incorporating data exchange standards and classification systems in e-business taxonomies”. Information Technology Management, XVI(4), 26-38.
P16. El-Diraby, T. E. Costa, J., and Singh, S. (2006). “How do contractors evaluate company competitiveness and market attractiveness: the case of Toronto contractors”. Canadian J. of Civil Engineering, 33, 596-608.
P15. El-Diraby, T. E., and Gill, S. (2006). "A taxonomy for construction terms in privatized infrastructure finance: toward a semantic cross-industry exchange of knowledge”. Construction Management and Economics, 24(2), 271-285.
P14. El-Diraby, T. E. and Zhang, J. (2006). “Constructability analysis of the bridge superstructure rotation construction method: the case of China”. Construction Engineering and Management, 132(4), 353-362.
P13. El-Diraby, T. E. (2006). “A web-services environment for collaborative management of product life cycle costs”. Construction Engineering and Management, 132(3), 300-313.
P12. El-Diraby, T. E. and Zhang, J. (2006). “A semantic framework for corporate memory management in building construction”. Automation in Construction, 15(4), 504-521.
P11. Lima, C., El-Diraby, T. E., and Stephens, J. (2005). “Ontology-based optimization of knowledge management in e-construction”. Information Technology in Construction (ITCON), 10(21), 305-327.
P10. El-Diraby, T. E., and Wang, B. (2005). “e-society portal: integrating urban highway construction projects into the knowledge city”. Construction Engineering and Management, 132(11), 1196-1211.
P9. El-Diraby, T. E., Lima, C., and Fies, B. (2005). “A domain taxonomy for construction concepts: toward a formal ontology for construction knowledge”. Computing in Civil Engineering, 19(3), 394-406.
P8. El-Diraby, T. E., and Brecino, F. (2005). “A taxonomy for outside plant construction in telecommunication infrastructure: supporting knowledge-based virtual teaming”. Infrastructure Systems, 11(2), 111-121.
P7. El-Diraby, T. E., and Kashif, K. (2005). "A distributed ontology architecture for knowledge management in highway construction". Construction Engineering and Management, 131(5), 591-603.
P6. El-Diraby, T. E., Abdulhai, B., and Pramod, K. C. (2005). “Integrated analysis of operation and feasibility of urban transit infrastructure: the case of Toronto monorail”. Canadian J. of Civil Engineering, 32(1), 58-71.
P5. El-Diraby, T. E., and O’Connor, J. T. (2004). “Lessons learned in designing research methodology in construction management field research”. Professional Issues in Engineering Education and Practice, 130(2), 109-114.
P4. El-Diraby, T. E., and Rasic, I. (2004). “Model for life cycle cost analysis of smart materials and intelligent devices”. Computing in Civil Engineering, 18(2), 115-119.
P3. Rivard, H., Froese, T., Waugh, L., El-Diraby, T., Mora, R., Torres, H., Gill, S., and O’Reilly, T. (2004). “Case studies on the use of information technology in the Canadian construction industry”. Information Technology in Construction (ITcon), 9(2), 19-34.
P2. El-Diraby, T. E., and O’Connor, J. T. (2001). "Model for evaluating bridge construction plans". Construction Engineering and Management, 127(5), 399-405.
P1. O’Connor, J. T., and El-Diraby, T. E. (2000). “Urban freeway bridge re-construction planning: the case of Mockingbird Bridge”. Construction Engineering and Management, 126(1), 61-67.
R13. Dorrah, D., and El-Diraby, T. E. (2018). “Mass timber high rise buildings”. Sidewalk Labs
R12. Piryoesi, M., and El-Diraby, T. E. (2018). “A Survey on Infrastructure Asset Management in Ontario”. Ontario Good Road Association.
R11. Piryoesi, M., and El-Diraby, T. E. (2017). “Data Analytics in Infrastructure Asset Management: Predicting Pavement Condition Index”. Ontario Good Road Association.
R10. El-Diraby, T. E., Kinawy, S. N., and Piryoesi, M. (2015). “Analyzing Approaches Used by Ontario Municipalities to Develop Road Asset Management Plans”. Ontario Good Road Association.
R9. El-Diraby, T. E. (2015). “A social-cyber-physical solution to crowd management in Haram”. Centre of Excellence in Transportation and Crowd Management, Umm Al-Qura University, Saudi Arabia
R8. El-Diraby, T. E., Karney, B., and Colombo, A. (2009). “Incorporating sustainability into infrastructure ROI: the case of leak management”. An Independent Study Funded by the Residential and Civil Construction Alliance of Ontario
R7. El-Diraby, T. E., Wolters, T., and Osman, H. M. (2009). “Benchmarking infrastructure funding in Ontario: towards sustainable policies”. An Independent Study Funded by the Residential and Civil Construction Alliance of Ontario
R6. El-Diraby, T. E. (2007). “Water and wastewater asset management in GTA: challenges and opportunities”, An Independent Study Funded by the Residential and Civil Construction Alliance of Ontario
R5. El-Diraby, T. E., and Osman, H. M. (2005). “Subsurface utility engineering in Ontario: challenges and opportunities”. Technical Report, Ctr. for Information Sys. in Infrastructure & Construction, U. of Toronto
R4. El-Diraby, T. E., and O’Connor, J. T. (1999). “System for evaluating bridge construction plans”. Technical Report, Ctr. for Transportation Research, Austin, TX.
R3. Harrison, R., Waldman, B. F., and El-Diraby, T. E. (1998). “Mitigating the adverse impacts of the Dallas North Central Expressway construction”. Technical Report, Ctr. for Transportation Research, Austin, TX.
R2. El-Diraby, T. E. (1996). “Decision making tools for construction engineers”. Special Report (also in Course material of CE395R-Decison & Risk Analysis - James T. O’Connor, Instructor), Civil Engineering Dept., University of Texas at Austin.
R1. Outland, D., Pearlson, K., El-Diraby, T. E., and others (1996). “GTE-Reengineering the customer response process”. Graduate School of Business, Univ. of Texas.
Key Projects
Mass Timber Modularization and Constructability
Team: Tamer El-Diraby and Marianne Touchie (PIs); Dalia Dorrah (graduate student)
This project was conducted in collaboration with Sidewalk Labs, Toronto.
This project investigates relevant issues for analyzing the feasibility and constructability of using mass timber in high-rise structures. New to the Ontario market, uncertainties and risks associated with such structures are under-studied. The motivation for adopting mass timber as the main structural element in high-rise buildings spans three dimensions. First, on the technical side, the key motivation for using mass timber structures is their positive contribution to energy management and resource conservation. Timber has a low embodied energy compared to concrete and steel. However, such an advantage can diminish in situations where timber has to be transported for long distances. Second, from a business perspective, exposed mass timber is a bold architectural feature that can be appealing to customers. In addition to the physical appeal, customers are becoming increasingly interested in supporting sustainable and locally-sourced material. A less obvious third dimension (or benefit) is to test and advance the resiliency and innovation capacity of the developer/contractor. The industry is poised for major changes due to factors that range from increased demands for sustainability, to the advent of smart building and artificial intelligence to, possibly the most important factor, the growing role of customers in decision making and co-creation of knowledge. Innovation and effective change of management abilities are keys to the competitiveness of developers and contractors in this emerging market. Taking on mass timber structures as a means to test and enhance organizational capacity for managing change and innovation can by itself be a good enough justification for such a decision.
Evaluation of related work and cases in the domain and the study of the main challenges for mass timber structures clearly indicate that detailed and collaborative analysis of the design and construction plans are essential to coordinating the innovative exploration and the successful execution of such new systems. Nevertheless, two issues of major significance need to be addressed. First, permitting and code compliance, particularly in regards to fire safety. Second, the newness of the system, which may be perceived as increase risk by the contractor. This is why, for the time being, mass timber structures in Ontario cost more. The increasing number of projects in Toronto should bring these costs to within range of regular structures. To this end, one of the key means for reducing the costs of mass timber is a collective effort by developers to streamline and strengthen the supply chain in Ontario—especially in relation to the manufacturing stage.
Investment in effective, open and interactive partnership with code agencies and research and testing institutes is one of the most important steps to be taken given the novelty and “unchartered” nature of such structures in Ontario. Code agencies will need sufficient testing/evidence and analysis before approving such structures. A dedicated program and/or group should be established to plan and continually update a collective effort to 1) synthesize and benchmark already existing knowledge and facts about the structural and fire performance of mass timber; 2) proactively commission testing, simulations and analyses to address any gaps or Ontario-specific needs; 3) consistently reflect the lessons learned in future projects; and 4) use smart, possibly IoT-based, monitoring systems to measure actual building performance, study and model behavior, and validate initial assumptions/simulations.
To address construction risks, developer and consulting teams should be actively engaged with the contractor. They should collaborate in modeling and sharing risks, training labour and supervisors, and monitoring productivity. In fact, owners should consider using the integrated project delivery system (IPD). This new practice creates a partnership between the owner and the contractor to share knowledge and work together to innovate, reduce risks, manage the project effectively, and, consequently, share the benefits and rewards. The use of BIM (Building Information Modeling) and virtual reality systems can be of great value to mass timber structure. First, virtual flythrough of the facility in 3D can be a key selling point to customers. Second, BIM can be used to visualize and create scenarios for the spread of fires and to study fire management plans and evacuation procedures. Finally, BIM can provide an effective platform for studying the project and co-managing its features (such as schedule and cost)
Survey of asset management practices
In collaboration with OGRA (Ontario Good Roads Assoc.), i2c researchers conducted two surveys for the asset management practices by Ontario municipalities.
Change management and policy making
i2c teams worked on two major areas for supporting competency of municipalities to conduct asset management; and in reengineering the environmental impacts assessment process to be more supportive of advanced knowledge and data management practices.
Report 1: competency for asset management
Report 2: reengineering the EIA process
Data analytics in asset management
In collaboration with OGRA, an i2c team used machine learning to predict road deterioration based on easy and free-to-collect data.
Report 1: FHWA report 18-065
Climate change and asset management
The above study was used to develop an interactive software to study the impacts of different climate change scenario on the future or road condition.
Social BIM
Through support from CANARIE, UofT team developed a BIM platform that integrates social and semantic network analyses and energy analysis to help educate and engage design/construction teams as well as end-users in optimizing the design and construction of green buildings.
Automated rule checking
I am working with buildingSmart International on building a framework for the consistent analysis of the business case for automated rule checking.
Data analytics in preliminary estimates
An i2c team is working with the Ministry of Transportation on using advanced data analytics to enhance the quality of preliminary estimates.
Initial budgeting and decision-making are based on planning cost estimates, which include a great deal of uncertainty. It is incumbent on engineers and planners to identify, and preferably quantify such uncertainties and communicate them to higher levels of management. Furthermore, they should assure the consistency of estimates throughout a statewide transportation plan. Consistency is important because the level of detail, hence uncertainty, is different for planning and project-level estimates.
Assessment of current practice at MTO: During preliminary design of projects, a budget estimate is needed to support decision making. Such estimates are developed based on the use of aggregate parametric analysis. Our first step is to review current conditions at MTO. This includes analysis of the process of early project estimation, including establishment of teams, setting assumptions, defining costing parameters, risk analysis and contingency planning. Furthermore, how the detailed estimation vary from preliminary analysis. This stage will include extensive interviews with MTO staff, including from estimation and construction departments as well as a sample of consultants. Of particular interest is to study approach to assigning allowance values for minor items, and means for assessing contingencies.
The main deliverable from this stage is a formalized Work flow of estimation process at MTO, including current rules of assessing risks and setting estimation parameters. Formalized description of gaps and needs as well as impacts of project scope on estimation accuracy. This stage will also include formalization of MTO staff expertise and their tacit knowledge about how to scope project delivery to enhance estimation accuracy and budget performance.
Data collection and quality: this project is reliant on data to help detect patterns of estimation scenarios and their association with project scope and risks: how the project configuration, typicality, and levels of details impact the accuracy of early estimation. Given the availability of data and the need for evidence-based decision making, data analytics could help transportation agencies utilizing their own data for smarter and more sustainable decision making. Processes that could benefit from data analytics include but are not limited to deterioration modeling, prioritizing maintenance and rehabilitations, risk management and project selection. Nowadays, other than collected technical data, many other sources of open data are available online for free. Combining these generic open databases with the technical databases could result in more appealing findings (Fox 2013). Data fusion could help taking into account the different facets of decision making problems and develop more robust machine learning models that can capture the complexity of data.
This stage will review existing project databases at MTO, including project sizes, early estimates, project conditions, work items, and context (for example, location and main design features). The dataset will be examined against MTO practices and with MTO staff to assess its representativeness. Furthermore, analysis will be conducted to compare data trends over the years: how things have changed over years and why?
Team
Ph.D. Students
1 Osman, Hesham, Cairo University, Egypt: Semantic modeling of infrastructure products, 2007
2 El-Gohary, Nora, University of Illinois, Urbana-Champaign, USA: Semantic modeling of integrated process management, 2008
3 Zhang, Jinyue, Tianjin University, China: Semantic modeling of actors and roles in construction, 2009
4 Abu Beih, Mahmoud, Accenture, UAE: Networked ITS systems, 2011
5 Nikbakhat, Mazdak, Concordia University, Canada: Social network analysis in infrastructure systems, 2015
6 Kinawy, Sherif, KPMG, Canada: e-society portal, 2016
7 Aragao, Rodrigo, Petrobras, Brazil: Network analysis for knowledge management, 2018
MASc. Students
1 Rasic, I.: Life cycle of smart infrastructure systems, 2002
2 Khan, K.: Ontology for highway construction, 2003
3 Gill, S.: Canadian experience in privatizing infrastructure, 2003
4 Pramod, K.: Feasibility analysis of a Toronto monorail, 2003
5 Zhang, J.: An ontology for building construction, 2003
6 Wang, B.: Taxonomy for sustainable infrastructure systems 2004
7 Abdelrahman, A.: Schema for infrastructure performance measurements, 2005
8 Surahyo, M.: Model for environmental costs of construction, 2005
9 Cariaga, I.: Marketing strategies in construction, 2005
10 Ballen, S.: Communication systems in construction, 2007
11 Bucci, M.: Analysis of environmental assessment practice in GTA, 2007
12 Zhang, Y.: Sustainability oriented feasibility model for construction, 2008
13 Illaszewiz, G.: Sensor networks in municipal water systems, 2008
14 Ismaili, D.: Evaluation of asset management competency, 2012
15 Mazen, I.: Business models for selling AEC knowledge over the Cloud,.2013
16 Isakov, B.: Engaging residents in building rehabilitation, 2015
17 Hosseini, M.: Social network analysis in transit systems, 2016
MEng. Students
1 Zhao, J.: Planning practices in waterway construction projects, 2002
2 Brecino, F.: Taxonomy for outside plant construction in telecommunication infrastructure, 2003
3 Ahmed, N.: Taxonomy for green concrete, 2005
4 Hanna, D.: Business models in Photovoltaic construction market, 2014
5 Chanana, S.: Data analytics for bid optimization, 2017
Ph.D. students
Piryonesi, Madeh: Asset management data analytics
Mahpour, Amir: Data analytics in project cost estimation
MASc. Students
Ng, J.: Data Analytics for building energy management
MEng. Students
Magarici, D.: Automated rule checking for intelligent buildings
Chawla, N.: Business model of street management in the smart city